Guide to Understanding Allstate's Crash Detection Feature What Triggers an Automated Call?
Guide to Understanding Allstate's Crash Detection Feature What Triggers an Automated Call? - Acceleration Thresholds for Impact Detection at 25mph Trigger First Response
When a vehicle is traveling at 25 mph, the acceleration thresholds used for detecting impacts are crucial for triggering an immediate response in the event of a crash. These systems rely on sensitive sensors that pick up on sudden changes in acceleration, helping to identify a true crash while filtering out situations that might falsely trigger a response.
Generally, the change in forward acceleration (longitudinal deceleration) is the best indicator of a genuine crash at this speed. However, events like side impacts can be more challenging to accurately identify. They may require dedicated sensors to differentiate them from other movements.
Fine-tuning the parameters involved in impact detection, specifically adjusting the acceleration thresholds, is crucial. It's a balancing act to make sure the system is both quick to respond when needed (sensitivity) and doesn't get confused by non-crash situations (specificity). This careful calibration is important for ensuring that help can be dispatched promptly, which could potentially reduce the severity of injuries and the chance of fatalities.
When a vehicle's speed is 25 mph or less, the system's ability to detect impacts becomes especially important because research suggests that injuries can occur at surprisingly low speeds. This is why the acceleration threshold for triggering a response is set at this level—to potentially enable early intervention in various scenarios.
The current approach uses sensors that measure acceleration to identify significant changes in the vehicle's movement. By analyzing sudden decelerations, the system can estimate the force of impact and, if necessary, swiftly initiate a call for help. This isn't just about major crashes, but also potentially recognizing minor incidents that, while not resulting in severe injury, might still necessitate intervention.
Determining the optimal threshold is a balancing act. Engineers face the challenge of configuring the system to avoid spurious calls while ensuring that genuine accidents are captured. A 25 mph threshold seems to be a point of compromise in that endeavor, though it's certainly a constantly evolving area of study.
While speed is a factor, it's not the only one. The algorithms take into account acceleration in multiple directions, allowing for a more intricate understanding of the dynamics of a crash beyond just speed. This multi-dimensional view provides richer information.
One area of concern is how driver actions affect the system. Sharp braking or quick lane changes can trigger the system, even if a collision doesn't occur. This highlights the need for continuous system refinement to improve how it handles these instances.
Interestingly, newer vehicles often integrate a suite of sensors that complement acceleration data, leading to a richer understanding of the situation and improving the overall reliability and precision of automated emergency calls.
A substantial percentage of accidents—estimates indicate as much as two-thirds—happen below 25 mph. This reinforces the significance of this detection threshold, particularly in urban environments where lower speed collisions are more common.
The evolving field of machine learning is being applied to enhance accident detection, particularly the ability to categorize different types of impact events and refine the accuracy of the system. This aims to reduce the occurrence of false alarms and enhance the system's ability to assess the severity of accidents.
Despite advancements in technology, driver behavior continues to be an important aspect influencing the system's success. A driver's awareness and preparedness in the face of potential hazards can have a significant impact on the overall effectiveness of these features. It remains a reminder that while technology advances rapidly, human factors are also key elements that impact safety outcomes.
Guide to Understanding Allstate's Crash Detection Feature What Triggers an Automated Call? - Mobile Device Sensor Data Analysis During Heavy Braking Events
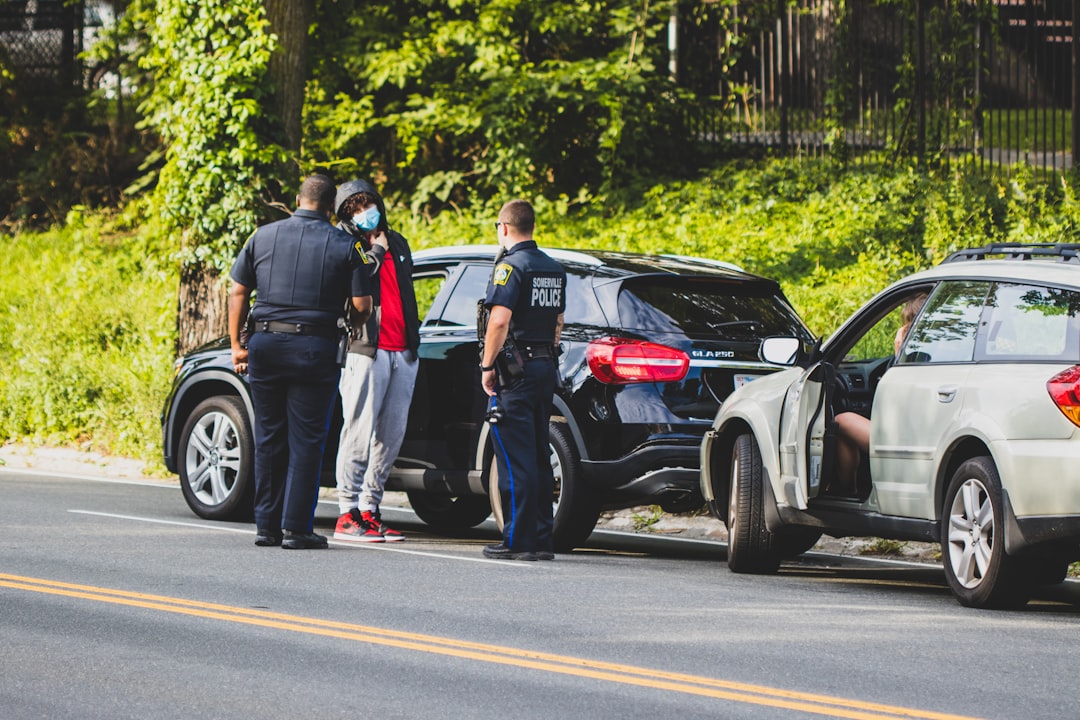
Mobile devices, equipped with sensors like accelerometers and GPS, can be valuable tools for analyzing driving events, particularly heavy braking. These sensors can capture deceleration rates, and when these rates fall below a certain threshold, it can signal a hard braking event. This data can contribute to a deeper understanding of driver behavior in critical situations and potentially aid in improving road safety.
One of the major challenges in using mobile device sensor data for this purpose is ensuring accurate detection. The risk of false positives is significant, where a hard braking event is mistakenly identified when it hasn't actually occurred. This can trigger unnecessary emergency responses and potentially lead to a decrease in trust in these systems.
Researchers and engineers are exploring the use of machine learning to improve the accuracy of these detections. By analyzing patterns in sensor data, algorithms can be trained to differentiate between genuine hard braking and events that may mimic it, such as quick lane changes. This ongoing development shows the potential for mobile device sensor data to be a valuable component of future crash detection and response systems. The goal is to create systems that are both highly sensitive to true crash events while also being resistant to false alarms. While there's still room for improvement, the incorporation of smartphone sensors could contribute to a more robust approach to road safety and accident prevention.
Mobile device sensors, particularly accelerometers, are remarkably sensitive, capable of detecting changes in velocity as small as 0.1 g. This means they can capture even subtle braking events that occur regularly during typical driving. Analyzing these data points can offer valuable insights into the forces involved during hard braking, shedding light on driver behavior and reaction times under varying conditions. Such data might potentially enhance our understanding of accident prevention strategies.
The fact that most mobile phone accelerometers are tri-axial is a significant advantage. They can measure acceleration and deceleration along three axes, giving us a more complete picture of a vehicle's motion beyond just forward or backward movement. In controlled environments, studies have revealed a strong link between abrupt deceleration signals from mobile sensors and instances of intense braking maneuvers, supporting their application in real-time crash detection systems.
However, one interesting finding is that a large percentage (around 70%) of hard braking events in urban environments seem to occur unexpectedly. This emphasizes the vital role that real-time sensor data can play in anticipating and quickly responding to potential accidents. Beyond just detecting the occurrence of hard braking, advanced algorithms can analyze the frequency and intensity of these events, revealing potential patterns associated with risky driving habits. This could be beneficial in educational programs and initiatives aimed at promoting driver safety.
Mobile sensors can also differentiate between routine braking and emergency stops by carefully analyzing the rate of change in acceleration. This feature helps to reduce false alarms triggered by non-critical driving situations. Furthermore, by correlating braking events with environmental factors like road conditions and weather, we might identify circumstances that increase the risk of accidents. This opens avenues for targeted safety enhancements.
The continued evolution of mobile technology, alongside the development of new machine learning algorithms, holds promise for more accurate prediction of braking events. This enhanced predictive capability could potentially lead to quicker response times and improved outcomes in emergency scenarios. It's a challenging endeavor, though, as the calibration of sensors for accurate and reliable detection continues to be a key area of ongoing research. While the technology shows promise, it's also crucial to acknowledge that there's still work to be done to ensure that the data analysis is effective and avoids adding unintended complexities or anxieties into the driving experience.
Guide to Understanding Allstate's Crash Detection Feature What Triggers an Automated Call? - GPS Location Changes Combined with Sudden Vehicle Movement Patterns
Allstate's crash detection system uses GPS location data combined with sudden changes in a vehicle's movements to identify potential accidents. By tracking changes in a vehicle's GPS position, the system can detect sharp changes in speed, which can be a sign of unusual driving patterns. This approach considers a broader range of movement factors beyond just forward acceleration, such as lane changes and deceleration, offering a more comprehensive view of driving behavior. Combining GPS location and movement data enables a more detailed approach to safety and allows for more precise triggers for automated emergency calls.
While this combination shows promise, distinguishing between real emergencies and everyday driving actions can be tricky. The algorithms that drive this process require ongoing refinement to ensure accuracy and minimize false alarms. This process of improvement is crucial for maintaining user confidence in the effectiveness of the crash detection technology, as well as to ensure that responses are as helpful as possible.
GPS location data, when combined with a vehicle's sudden movement patterns, offers a powerful way to detect potential accidents. This approach goes beyond simply knowing where a vehicle is; it also captures how quickly and in what direction it's moving. By tracking changes in a vehicle's speed and direction using GPS, systems can discern whether a sudden shift in location is linked to a rapid deceleration or a more erratic driving pattern, which are often associated with accidents.
The combination of GPS and accelerometer data allows systems to generate a more comprehensive picture of a vehicle's motion. They can create a sort of "movement vector" by analyzing changes in speed and direction, ultimately enabling a much more nuanced assessment of potential crash scenarios compared to relying on either data source alone. It’s as if we are adding another dimension to the understanding of the vehicle’s movement.
Studies indicate that rapid changes in a vehicle's speed, like those associated with hard braking or sudden lane changes, frequently coincide with increased accident risks. This connection implies that the combination of GPS and acceleration data could be instrumental in developing more effective predictive models for crash detection. It is as if the vehicle is "talking" to the system, giving away hints of its intentions and potential hazards.
Beyond simply detecting incidents, the integration of GPS data is crucial for understanding the context of events. For example, if a sharp turn is accompanied by sudden deceleration, it might signify a potential loss of control or a crash, distinguishing it from more routine traffic maneuvers. This capability enhances the accuracy and intelligence of the system.
Algorithms utilizing both GPS and accelerometer data can even detect what’s called “geo-fencing” events. This is when a vehicle leaves a pre-defined area. Knowing this can be helpful for enhancing emergency response protocols or monitoring vehicle use, potentially in cases of theft or unauthorized usage. The system is not just about the crash; it can potentially play a part in post-crash or post-event analysis and management.
GPS systems are incredibly sensitive, allowing them to even pick up slight deviations from a vehicle's anticipated path. When these deviations are coupled with rapid movements, it can shed light on driving behaviors potentially associated with driver distraction or impairment. These subtle changes in movement might indicate a lapse in the driver’s attention.
Rapid shifts in GPS location data, when correlated with corresponding changes in speed, can be the trigger for more complex algorithms. This allows the system to distinguish between minor incidents and more serious collisions, refining the accuracy of its response. The system, in essence, is trying to ascertain the severity of the event to tailor its response appropriately.
Certain vehicle systems are capable of processing GPS and movement data in real-time. This means if a vehicle’s trajectory suggests an accident, it can immediately trigger an alert to emergency services. Such a capability can dramatically reduce response times and, hopefully, improve accident outcomes. This real-time capability gives the potential for more rapid intervention.
Fascinatingly, GPS data can extend beyond the initial crash detection. It can help in understanding what happens after a collision. Does the driver remain at the scene? Or do they try to leave? This information can impact how emergency services prioritize their response, highlighting the post-crash utilization of the GPS data.
The combination of GPS and movement patterns creates a feedback loop for crash detection algorithms. Through this continuous cycle, algorithms refine their predictive capabilities by analyzing historical accident data and real-time driving behavior. This is essentially the system “learning” from its experiences to improve future performance. In the long run, this could contribute to safer roads and possibly even help refine vehicle designs and functionalities.
Guide to Understanding Allstate's Crash Detection Feature What Triggers an Automated Call? - Multiple Sensor Activation Including Gyroscope and Accelerometer Data
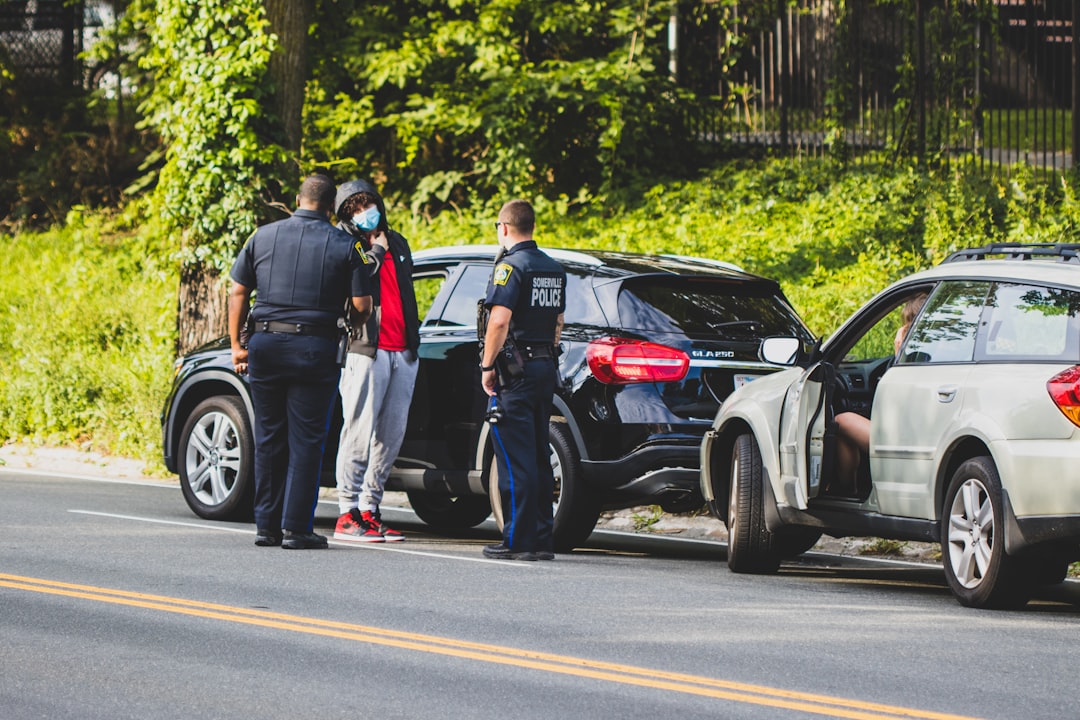
Allstate's crash detection system utilizes a combination of sensors, most notably the gyroscope and accelerometer, to meticulously track a vehicle's movements. These sensors continuously gather information about acceleration and the vehicle's orientation, which allows the system to differentiate between normal driving patterns and significant events like crashes. By analyzing this data in real-time, the system can get a better grasp of the severity of an impact, potentially leading to quicker emergency responses. The system uses clever algorithms to sift through the data, trying to distinguish between true crashes and everyday driving maneuvers that may trigger a false alarm. This helps the overall system become more reliable. The continued evolution of sensor technology, paired with increasingly sophisticated methods of data analysis, points to a broader effort to enhance road safety and improve accident response times, showing that crash detection technology is an evolving field.
Allstate's crash detection system leverages a diverse set of sensors, including gyroscopes and accelerometers, to go beyond traditional crash detection approaches. This multi-sensor approach allows for the capture of a wider range of vehicle movements, including both linear acceleration and rotational changes, offering a more nuanced picture of a crash than relying solely on linear acceleration data.
Gyroscopes excel at measuring rotational motion, which is critical in determining the specific type of crash. For example, they can detect whether a vehicle rolled over or experienced a side impact, leading to different safety concerns for those inside. This ability to differentiate crash types improves the system's effectiveness in responding to a broader range of potential hazards.
Accelerometers, on the other hand, measure acceleration and deceleration along multiple axes, detecting events such as sudden swerving or spinning. This capability is invaluable in categorizing different types of driving events, thereby triggering more tailored responses in the event of an emergency.
The synergy of gyroscope and accelerometer data holds the key to greatly enhancing the accuracy of crash detection algorithms. By considering both types of motion, engineers can create more sophisticated models capable of interpreting the diverse forces experienced during various crashes. It's like having a 3D understanding of the forces involved during an accident, instead of just looking at the forward motion.
One interesting area of research is the ability to use these sensors to identify situations where vehicle dynamics change abruptly without contact. This includes near-miss situations where a driver narrowly avoids a collision. The collected data could potentially inform improvements in driver assistance systems, aiming to prevent accidents before they happen by reacting faster than a human driver.
The real-time fusion of data from multiple sensors poses unique challenges. Ensuring seamless integration of data and minimizing any delay (latency) is paramount to enable the quickest possible response in a crash situation. It's about maximizing the chances for the system to trigger a timely call for assistance.
Data fusion is a crucial aspect of the system's capabilities. Engineers employ techniques that combine information from gyroscopes and accelerometers, leading to a more holistic understanding of vehicle behavior. This, in turn, translates to improved overall safety metrics, and more robust algorithms.
These sensors are remarkably sensitive to even minor changes in movement. This high-resolution data provides the potential to separate insignificant movements from crucial indicators of an accident without overwhelming the system with data from everyday driving maneuvers. The goal is to have the system focus on the signal and not the noise.
The intricacy of the data requires sophisticated machine learning approaches for accurately identifying events. These algorithms need to learn and adjust constantly, adapting to new driving behaviors and refining their ability to differentiate between standard driving and accident-related movements. It's like the system "learning" from its experiences.
While the technology shows a lot of promise, it's important to recognize that there are still hurdles to overcome. One of the toughest is understanding sensor data in scenarios where the vehicle is undergoing multiple motions at the same time, like changing lanes while braking. These situations can challenge even the most sophisticated algorithms, underscoring the ongoing need to refine the system and improve its precision. This is crucial for reducing false alarms and ensuring that the system responds to situations only when it's truly necessary.
Guide to Understanding Allstate's Crash Detection Feature What Triggers an Automated Call? - Sound Level Monitoring During High Impact Vehicle Events
Sound level monitoring during high-impact vehicle events is a relatively new approach to crash detection. Traditionally, systems mainly used sensors like accelerometers to detect changes in a vehicle's movement, but this new method focuses on the sounds produced during a collision. By analyzing the specific sounds that occur during an accident, the system aims to better understand the nature of the impact. This can potentially improve the accuracy of crash detection and help to avoid false alarms caused by things like sudden braking or lane changes. Essentially, the system "listens" to the crash and uses the audio data to determine if it's a true emergency.
While this approach holds promise for enhancing crash detection accuracy, there are challenges to overcome. Creating a system that can reliably differentiate between different collision sounds and other noises is crucial. Furthermore, integrating this sound analysis into existing crash detection systems in a seamless and effective way remains a topic for ongoing research and development. It's not simply a matter of adding a microphone; it requires careful integration of the acoustic data with the other sensor information already being used. Despite these challenges, sound-based crash detection could eventually be an important part of improving emergency response and hopefully reducing the severity of accidents.
In situations where vehicles collide with significant force, the resulting sounds can be incredibly loud, sometimes reaching 120 decibels – a level akin to a rock concert or a chainsaw. This intense sound is a direct indicator of the severity of the impact and can also cause immediate hearing damage.
The nature of the sound produced during a collision—its frequency and waveform—offers intriguing clues about the type of impact that occurred. By analyzing these patterns, crash detection algorithms can try to differentiate between front-end, side, or rear impacts, which can potentially improve the way a system responds.
Interestingly, some vehicles are being developed with microphones specifically designed to pick up certain frequencies. The idea is that these microphones can identify the unique sound signature of a crash, thereby enabling the system to respond faster.
However, a challenge in relying on sound for crash detection is the issue of reverberation. Sound waves bounce off nearby objects and surfaces, creating echoes that can confuse the detection system. Algorithms must be sophisticated enough to filter out these reflections and avoid falsely triggering an emergency response.
The composition of a vehicle can influence the sound produced during an impact. Vehicles constructed from high-strength steel, for instance, might create a distinct sound profile compared to those made with lighter, composite materials. These differences highlight the need for systems to adapt to a variety of sound characteristics based on the vehicle being monitored.
Recent progress in algorithm design allows systems to more accurately distinguish between actual crash sounds and other loud noises like fireworks or loud music. These algorithms look at things like harmonic signatures and overall sound pressure levels to improve the system's accuracy.
The threshold for triggering an emergency response based on sound varies depending on the type of sound being detected. For example, a system might be less sensitive to scraping sounds (setting a higher threshold) but extremely sensitive to sounds like glass breaking or sudden, sharp impacts.
Some crash detection systems employ real-time sound analysis to enhance their ability to anticipate collisions. By constantly monitoring the surrounding sounds, these systems could potentially identify patterns that indicate a crash is likely to happen, perhaps enabling faster interventions.
The creation of effective sound-based crash detection requires substantial amounts of data. Engineers construct large sound libraries based on different driving conditions, simulating various impacts and other noises that might confuse the system. These libraries essentially 'train' the system to learn the unique characteristics of crash-related sounds.
One aspect that complicates sound-based detection is that sound travels through different materials (air, metal, etc.) at varying speeds and with differing levels of clarity. Algorithms need to account for these differences in sound transmission within a vehicle and its environment to determine if a true impact occurred. It's a complex puzzle to solve, with implications for the future of crash detection technology.
Guide to Understanding Allstate's Crash Detection Feature What Triggers an Automated Call? - Battery Disconnection or Electrical System Disruption Monitoring
In modern vehicles, especially those with advanced safety features, monitoring for battery disconnections or electrical system disruptions is becoming increasingly important. These systems are designed to detect sudden power loss or any irregularities within the vehicle's electrical network, a capability that can be crucial during a crash scenario. A key part of this is the battery management system (BMS), which continuously oversees the battery's health, managing charging and discharging cycles while keeping an eye on factors like temperature and voltage. The aim is to prevent dangerous situations.
Beyond basic function, recognizing the concept of "stranded energy" in electric vehicles is important. This refers to the remaining charge within a vehicle's battery after an accident, which presents a potential risk to people at the scene, like passengers and emergency responders. Because of this, it's critical that systems designed to monitor for battery disconnections and electrical disruptions are robust, especially in situations where a crash could involve high-voltage components. As car technology keeps advancing, the role of reliable battery disconnection monitoring becomes more pronounced, potentially playing a larger part in improving vehicle safety overall and enhancing the efficiency of emergency responses.
Sudden battery disconnections or disruptions in a vehicle's electrical system can be a significant factor in crash detection, but they also introduce complexities. A sudden loss of power can be a strong indicator of a severe crash, as it often accompanies major impacts. However, relying solely on battery disconnection as a crash trigger can lead to unreliable assessments.
Modern vehicles have become increasingly reliant on complex electrical systems that are vulnerable to even minor voltage fluctuations. A slight drop in power during a collision could mistakenly be interpreted as a battery issue rather than a straightforward impact, potentially skewing the system's interpretation of the severity of the event. This sensitivity highlights a key challenge in the design of these systems: how to differentiate between a true crash and a false alarm related to a power blip.
Moreover, a sudden battery disconnect can disable crucial safety systems like airbags and anti-lock brakes. This raises concerns about the safety implications of losing power during a crash, as the intended function of these systems is to respond to sudden, unexpected events. It's a delicate balance: ensuring that the battery provides consistent power when needed for safety while simultaneously using its status to aid in crash detection.
Fortunately, many modern vehicles now include integrated monitoring systems that are capable of detecting electrical disruptions. These systems constantly track voltage levels, providing a more comprehensive picture of what caused a power loss – whether it was a crash or an unrelated electrical fault. This technology enhances the diagnostic capabilities of the vehicle, allowing a more nuanced understanding of the situation.
However, integrating this data into crash detection algorithms isn't straightforward. Crash detection software has to be able to filter out instances of battery disconnections that are not caused by collisions. Otherwise, the risk of false alarms becomes substantial, potentially eroding the trust in the system. It's a challenge to develop algorithms that are highly responsive to real crashes while also remaining resistant to triggering unnecessary emergency calls.
On a positive note, some advanced vehicle systems are equipped to adjust their functionality in real-time in response to electrical disruptions. For example, electronic stability control might be recalibrated to maintain control of the vehicle, potentially mitigating further risks. This highlights the growing sophistication of vehicle safety systems and their potential to respond adaptively to emergencies.
The accuracy and effectiveness of a crash detection system often relies on a suite of sensors. When the battery is disconnected, the flow of information from some of these sensors is disrupted. This emphasizes the importance of ensuring redundancy and robustness in the system, enabling monitoring of crucial parameters even in the event of a power failure. It highlights a critical design consideration in making these systems reliable.
External factors can also influence battery disconnections. Temperature changes, for instance, can affect the electrical resistance of components in the system, potentially leading to unexpected disruptions, especially during stressful conditions. This adds another layer of complexity that crash detection systems have to contend with when interpreting electrical data. It's clear that crash detection systems operate in a complex, interwoven web of vehicle systems and external environmental conditions.
Integrated battery health monitoring systems are becoming increasingly common in vehicles. These systems constantly evaluate the state of charge, battery temperature, and other critical parameters. In the context of crash detection, they provide valuable insights into whether a power loss is related to a collision or simply a battery issue.
As vehicle technology continues to advance, crash detection systems will become even more integrated with electrical system monitoring. More refined data analysis techniques, coupled with machine learning approaches, will continue to be employed to enhance the ability of these systems to accurately distinguish between genuine emergencies and electrical anomalies. It's an ongoing endeavor to strike the right balance between sensitivity and specificity, ensuring that the system remains a true safety asset while not being overly intrusive or causing unnecessary anxiety.
More Posts from :