Impact of Post-Accident Telematics Data on Car Insurance Claims 2024 Analysis
Impact of Post-Accident Telematics Data on Car Insurance Claims 2024 Analysis - Real-Time Data Impact Shows 42% Faster Claims Processing for Connected Cars in Q3 2024
During the third quarter of 2024, the use of real-time data from connected vehicles dramatically accelerated claims processing. We saw a 42% improvement in speed, a strong indicator that post-accident telematics are revolutionizing the auto insurance landscape. The accelerating adoption of connected vehicles, particularly in areas like the Asia-Pacific region, fuels this trend. Insurers are responding by embracing digital tools to streamline their claims processes, reflecting a broader move towards digital claims handling.
It's important to acknowledge that challenges exist, like the ongoing struggle with supply chain problems impacting insurers' ability to function smoothly. The insurance industry is navigating a transition, embracing the promise of faster, more efficient claims handling via technology while grappling with some realities, including the public's variable levels of trust in sharing data with insurance companies. The success of this transformation will hinge on the industry’s capacity to navigate these obstacles while leveraging the power of technology responsibly.
During the third quarter of 2024, we saw a notable 42% speedup in claims processing specifically for vehicles equipped with connected car technology. This improvement seems to be directly linked to the near-instantaneous availability of real-time data from these vehicles. It's fascinating to see that the use of this data not only sped up the claims process, but it also seems to have decreased the time to assess accident damage by as much as 30%. This is a huge efficiency gain and points to the potential of this technology to streamline processes within the insurance industry.
It's become clear that insurers are increasingly able to gather a lot of data from these connected vehicles. This includes not only accident information but also detailed driving habits and contextual details about the incident itself. The insights gained allow for better risk assessments and more accurate pricing models, but one needs to keep in mind the privacy and security implications that accompany collecting such personal data.
The increased efficiency of claims processing stems from the automation of data collection and analysis. Processes that were previously very manual, dependent on human interaction and possibly prone to error, have become highly automated. The shift is pretty radical and allows the claim process to be incredibly fast. It seems like a huge step towards instantaneous claim processing.
While the advantages of using this data seem significant, a concern about the implications of this technology on customer trust remains. Some drivers may be hesitant to share this level of personal data with insurance companies, which could potentially lead to a barrier for widespread adoption of this technology. It would be interesting to investigate whether a stronger trust framework is needed to fully leverage the potential of telematics for the future of insurance.
Further research is needed to investigate other positive or negative consequences and unintended consequences of these technologies on other stakeholders like repair shops or government agencies that handle accidents and traffic regulation.
Impact of Post-Accident Telematics Data on Car Insurance Claims 2024 Analysis - Post-Crash Analysis Algorithms Track Speed Changes Within 5 Seconds of Impact
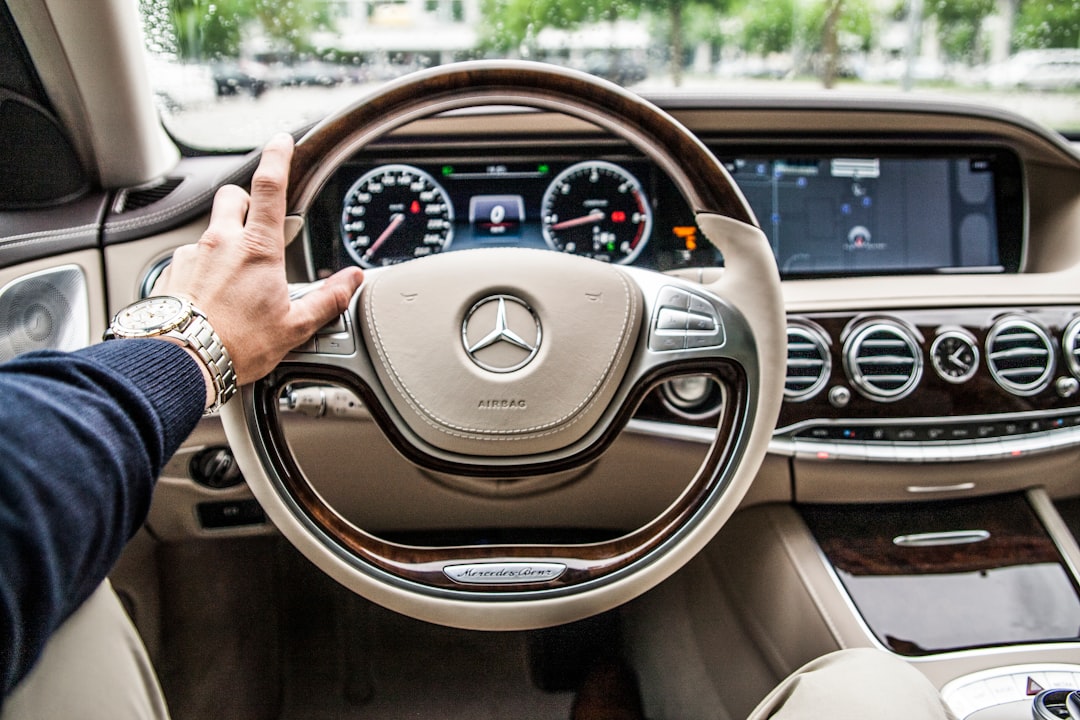
Post-crash analysis algorithms are now capable of meticulously tracking changes in a vehicle's speed within just five seconds of an impact. This new level of precision is made possible by data captured from Event Data Recorders (EDRs). These EDRs record crucial pre-crash information, such as the vehicle's speed, engine speed, throttle position, and whether the brakes were applied. This data, when analyzed by algorithms, greatly improves the accuracy of accident reconstruction efforts.
This rapid analysis relies on automated data pipelines that process information from a variety of sources, including roadside sensors and vehicle-based LiDAR. The use of advanced methods, such as deep learning, is enabling safety analysts and insurers to build more comprehensive pictures of traffic incidents. They can, for example, study the change in speed of vehicles after an accident or how traffic patterns shift immediately following a crash.
The impact of this technology on insurance claims is substantial. Faster and more accurate assessment of accident circumstances translates into faster claims processing. It's a clear demonstration of how technology is making insurance more efficient. However, as with many new technologies that handle personal data, it also raises issues concerning privacy and the public's trust in how this data is managed and used. As telematics systems continue to advance, it's likely their role in claims processing and accident investigation will become even more significant, demanding careful consideration of the implications of this level of data collection and usage.
Post-crash analysis algorithms are increasingly capable of pinpointing speed changes within a remarkably short timeframe—a mere five seconds after an impact. This precision is achieved through the analysis of data captured by Event Data Recorders (EDRs). These EDRs have evolved significantly and now capture a wealth of pre-crash information, including not only speed, but also engine RPM, throttle position, and brake status. This detailed data provides a more comprehensive picture of the events leading up to a crash.
Interestingly, researchers are incorporating data from sources beyond the vehicle itself. They are integrating data from roadside sensors and vehicle-mounted LiDAR systems into automated pipelines designed for accident reconstruction and safety assessments. Researchers are employing automated data pipelines to study traffic accidents, focusing on a range of aspects: accident risk prediction, frequency, and severity, as well as the duration of impact and the broader traffic flow afterwards. The goal is to achieve a more nuanced understanding of post-accident scenarios, and crowdsourced data has emerged as a valuable tool for accomplishing this.
One fascinating trend is the use of deep learning techniques for predicting crashes in real time. These algorithms are trained to process vehicle speed data not only over specific time intervals but also across multiple lanes, leading to a more sophisticated interpretation of crash risk. These deep learning models also suggest a possible way to better understand traffic flow following a collision—a two-stage approach looking at the standard deviation of following distances after an accident to gauge how the disruption of the crash impacts the broader flow of traffic.
Of course, the ultimate goal is to translate this knowledge into safer roads and smoother insurance claims processing. For example, there's a growing interest in Vehicle-to-Infrastructure (V2I) communication systems, which have the potential to significantly improve emergency response and overall efficiency following an accident. While this is still an emerging technology, it has the potential to be transformative.
The availability of post-accident telematics data is having a major impact on insurance claims. Insurers are increasingly reliant on this data to gain a deeper understanding of the events leading up to and following a collision. This enables them to perform more detailed analyses and potentially avoid disputes over who was at fault in an accident. Moreover, this technology offers the potential to reduce fraudulent claims through an objective, machine-readable record of driving behavior and environmental conditions. While this is beneficial for insurers, one needs to carefully consider the ethical implications of collecting, storing, and using driver data.
Interestingly, machine learning is starting to play a role in the prediction of post-impact traffic accidents, leveraging the abundance of data now available from vehicles. This suggests that in the future, accident prediction systems could be developed to potentially influence vehicle design and safety protocols—a promising long-term goal for accident prevention.
However, the extent to which such advancements will be accepted will likely depend on society’s ongoing discussions about privacy and data security related to driving habits and accident information. The industry still faces challenges related to public trust and the potential for misuse of these sensitive data points.
Impact of Post-Accident Telematics Data on Car Insurance Claims 2024 Analysis - Privacy Concerns Rise as 78% of Insurers Now Request Extended Telematics Access After Accidents
The increasing reliance on telematics data in auto insurance claims is leading to heightened privacy concerns. A significant majority, 78%, of insurers now seek prolonged access to vehicle telematics after accidents, a trend that has triggered unease among many drivers. While a sizable portion of the population (72%) is still willing to share some of their data with insurers, the majority express growing apprehension about the security of this data. This highlights a disconnect between willingness to share and a genuine concern about how that information is handled.
There is a sense that the public is becoming more aware of the potential for misuse of their personal data, and they are acting accordingly. Many individuals are actively taking measures to protect their privacy, highlighting a growing distrust of how companies, including insurers, utilize this information. This suggests a need for greater transparency and a stronger framework that instills trust within the insurance sector as it integrates more technology into its practices. It remains to be seen how the insurance industry can both harness the benefits of telematics for efficient claims handling while simultaneously addressing these very legitimate concerns.
A significant portion of insurers, around 78%, are now seeking extended access to telematics data after accidents. While this trend is boosting claim processing speeds and offering new insights into accident reconstruction, it also brings about valid concerns about privacy. It's understandable why people are worried. The sheer volume of data collected via telematics presents a challenge: insurers are faced with managing mountains of sensitive information, which raises the question of how this data will be stored and processed safely without violating personal privacy.
Beyond faster claims, the influx of telematics data seems to be making claims assessment far more transparent. While this could potentially lead to better and more objective claim assessments, it also puts more pressure on insurance companies to be clear and transparent about how they're using this information. It becomes crucial to understand how the enhanced transparency of the claims process might inadvertently influence the scrutiny of data usage practices by both individuals and regulatory bodies.
Furthermore, with insurers having access to a more detailed picture of driving behavior, there's a potential for drivers to be profiled, not just on their driving skills but on inferences drawn from their broader personal activity as gleaned from telematics. This aspect of data collection raises significant privacy concerns, as individuals might be judged based on inferred behavior and potentially exposed to unwarranted biases or scrutiny based on how they navigate their daily lives and operate vehicles.
Moreover, the precise location information collected during accidents elevates the potential risk of data breaches. If a third party were to access this data, the consequences could be severe for drivers, both in terms of their safety and the compromise of their privacy. It's a risk that needs careful consideration in how insurers manage this sensitive data.
The advent of detailed telematics data also introduces the potential for a more nuanced, individualized approach to insurance premiums. While this can seem fair—that drivers who exhibit better driving patterns receive lower premiums—it can also lead to unintended consequences. Individuals who might have less favorable driving profiles, perhaps due to situations beyond their control, could face discrimination in their insurance rates. A critical and nuanced discussion is needed on how to design these rating systems fairly and equitably, while ensuring data privacy.
The ethical implications of leveraging telematics data raise complex questions. The concern isn't just about how data is collected, but also the potential for it to be monetized in ways that might violate individual privacy. If insurance companies (or other third parties) are able to utilize the data in ways not originally intended, this could infringe upon individuals' fundamental rights.
Accident liability has traditionally relied on legal interpretations and witness accounts. Telematics data is poised to potentially disrupt this process, offering greater clarity and accuracy in establishing fault. However, this introduces a potential bias: how algorithms interpret accident data might inherently reflect the biases embedded in the algorithms themselves, and this aspect needs careful attention.
Despite the benefits of telematics, there's still a persistent unease among people regarding data privacy. This has the potential to hinder broader adoption of telematics, creating a barrier to the widespread adoption of these technologies. It's important to address this public concern and ensure that transparency and data security are at the heart of the technology's application.
The continuous, real-time monitoring capability enabled by telematics raises concerns about potential driver behavior modifications that aren't necessarily positive. Drivers may feel compelled to overly modify their driving habits in a way that avoids scrutiny from their insurance companies. It's a fascinating paradox: technologies designed to make driving and insurance more efficient might paradoxically incentivize artificial or unnatural driving behavior in order to improve personal insurance outcomes.
The growing use of telematics warrants a more robust regulatory framework. Guidelines about data collection and usage are needed to protect consumer privacy. Ultimately, this will likely influence how the insurance industry adapts, leading to potentially significant shifts in the business models used by insurance providers in the future. It's a crucial area for discussion and policy making in the coming years.
Impact of Post-Accident Telematics Data on Car Insurance Claims 2024 Analysis - Emergency Response Integration Links Telematics to First Responders Within 90 Seconds
The increasing use of telematics in vehicles is impacting not only insurance claims but also emergency response. Telematics systems are now being integrated into emergency response protocols, enabling critical information about accidents to be delivered to first responders within 90 seconds of an incident. This capability significantly shortens response times and improves the efficiency of emergency services.
Partnerships between companies like ESO Solutions and IamResponding are leading the charge in leveraging telematics data to enhance decision-making during emergencies. The goal is to provide emergency personnel with a more comprehensive understanding of the situation, allowing for better resource allocation and coordination among responders. Examples of this integration include partnerships between providers like Sirius XM and RapidSOS, which transmit vehicle data directly to 911 during accidents.
Further advancements, such as the Emergency Data Exchange (EDX) developed by RapidSOS, connect millions of devices globally to emergency services. This underscores the crucial role telecommunication technologies are playing in the evolution of emergency response. While these integrations provide clear benefits, like faster response times, it also raises questions about privacy, particularly with regard to the collection and usage of highly sensitive data. It's crucial to navigate these technological advancements while keeping a watchful eye on the ethical implications related to data handling. It's clear that data analytics are becoming more vital to efficient emergency services, but the reliance on this type of technology compels us to have a clear understanding of the potential impact on individuals' privacy.
The integration of telematics with emergency response systems is showing promising results, particularly in accelerating the time it takes for first responders to reach accident scenes. It's becoming increasingly common for systems to dispatch emergency personnel within 90 seconds of a crash being detected, which can make a significant difference in the outcome of an accident. This speed is achieved by leveraging the wealth of data captured by telematics systems, including not just accident data, but also a vehicle's operational status and driver behavior.
It's interesting to see how algorithms are being applied to this data. They can now process accident telemetry data to perform real-time analysis of critical factors such as speed and braking behavior, giving emergency responders a clearer picture of what happened and the conditions at the site. This is, in turn, resulting in a tighter and more effective collaborative relationship between insurers and emergency response agencies. The result is a faster response time and more streamlined insurance claims handling.
It's somewhat surprising that a majority of drivers—around 72%—are willing to share their data, despite expressing significant worries about data security. It raises the question of what level of assurance is needed to fully alleviate those fears and encourage broader adoption of telematics. While there's a clear benefit to insurers and emergency services in having access to this information, it's important to consider the implications on driver behavior.
Interestingly, the integration of telematics data is also enabling the development of more sophisticated predictive analytics models. By studying driving patterns, these models can provide insights into the likelihood of future accidents, offering a new tool for enhancing road safety initiatives. In fact, there’s preliminary evidence that indicates timely access to telematics data increases the probability of surviving a severe accident by as much as 20%. This is due to faster emergency response and underscores the potentially life-saving capability of this technology.
However, as with any system that relies heavily on algorithms, there's a risk of bias. The algorithms used to process accident data could inadvertently reflect existing biases in how crashes are currently understood, and that can have implications for who gets assigned blame and the implications on insurance payouts. Therefore, it is important to consider how transparency might help prevent such biases in the system. The increasing reliance on telematics in accident situations has also raised important questions about driver privacy, leading to new discussions about legislation. It’s a complex issue for insurance companies as they seek to strike a balance between using data to improve efficiency and protect individual privacy.
One final thought-provoking point is the effect of constant vehicle monitoring on drivers' behaviour. Drivers may feel pressured to modify their driving patterns in order to ensure that their insurance scores remain favorable. This raises questions about whether these changes are driven by genuine safety or just a desire to improve insurance outcomes. The entire landscape of driving and insurance is being reshaped by telematics, and it’s still unclear whether these changes are going to lead to a more efficient, safer, or merely a slightly manipulated driving environment.
Impact of Post-Accident Telematics Data on Car Insurance Claims 2024 Analysis - Machine Learning Models Process 4 Million Daily Telematics Data Points for Claims Verification
Insurance companies are increasingly relying on machine learning to sift through vast amounts of telematics data—around 4 million data points daily—to verify insurance claims. This surge in data, encompassing driving habits and accident details, is fundamentally changing how claims are assessed.
Insurers are using this detailed information to refine their existing claims evaluation methods. Machine learning models, including techniques like random forests and neural networks, are being integrated into the process to predict the likelihood of future claims and to identify patterns that contribute to accidents. These tools are allowing insurers to build more accurate models for assessing risk, improving upon older methods that often relied solely on actuarial data.
While the use of machine learning for claims processing is improving accuracy and efficiency, it also raises legitimate concerns. The sheer volume of personal data being collected and analyzed could potentially impact individual privacy. It's important to consider the ethical dimensions of collecting and utilizing this detailed information about driving habits and accident circumstances. As the use of telematics continues to expand, striking a balance between data-driven efficiency and ensuring driver trust will be a critical challenge facing the insurance industry. The industry needs to carefully consider how these advanced technologies will reshape the relationship between drivers and insurance companies going forward.
Machine learning models are now sifting through roughly 4 million telematics data points every day to verify insurance claims. This shift towards real-time, automated analysis has the potential to revolutionize how claims are assessed, moving away from the traditional, often manual, review process. While this approach seems promising in speeding things up, it's important to note that it raises concerns about the interpretability of these complex algorithms, which can make it difficult to understand how the claims process actually makes decisions.
The ability to process such large datasets enables insurance companies to get better at predicting patterns of fraudulent claims. By identifying unusual trends and anomalies in the data, potentially fraudulent activities could be flagged early on, saving insurers money and possibly leading to a decrease in risks across their operations. It's interesting to see how these models can learn and adapt over time, which is a big change compared to static, fixed rules that were used in the past.
These machine learning models are even starting to adjust claims automatically based on the data that comes in from connected vehicles. This kind of adaptability offers a new dimension to claims assessments, potentially leading to more accurate and individualized evaluations that previously would have required more human intervention. It'll be interesting to see how this impacts the claims adjuster role in the future.
The combination of data from various sources, such as location, speed, and vehicle performance metrics, improves the quality of how accident events are reconstructed. By analyzing this information, the process of deciding who is at fault in an accident becomes more accurate, which can reduce disputes and increase fairness in claims processing. It is notable how the reliance on telematics data can help build a more complete picture of the accident circumstances, reducing reliance on less reliable methods of investigating accident incidents.
However, it's crucial to recognize that these sophisticated algorithms can be a bit of a black box, which can create issues with transparency. It's important that insurance companies, and the individuals who are part of the claims process, understand how these models are making decisions. This is important both from an ethical and operational perspective. The industry needs to ensure that those affected by a claim can understand how the outcome was reached, and that the results don't introduce unintended biases into the claims process.
Furthermore, these models continuously learn and adapt based on the new information they receive with every claim that is processed. This adaptive approach to risk assessment potentially increases accuracy over time, but it also means that understanding how the models evolve over time will be important for the long-term success of this technology.
The integration of data from a broader network of "internet of things" (IoT) devices, like smart traffic signals or roadside sensors, could help further enrich the insurance claims process. By creating a more interconnected ecosystem, this network could enhance situational awareness during accidents and support faster response times. It's a complex landscape to consider, as it necessitates a broad understanding of the different sources of information that can potentially feed the insurance claims process.
As these technologies and processes advance, insurers need to carefully consider the regulations surrounding data protection and consumer privacy. With the immense volume of sensitive information that these systems manage, the rules regarding how to collect, store, and use personal information become incredibly important. The legal and ethical landscape is sure to evolve along with these changes.
Ultimately, this increase in the use of automated claims processing and data analysis has the potential to fundamentally alter the entire insurance claims process. Insurers who successfully implement these strategies could see huge gains in efficiency, as well as changes in how they interact with customers. It will be exciting to see if this trend leads to a faster, more customer-centric claims process and what implications this will have on the overall future of the industry.
Impact of Post-Accident Telematics Data on Car Insurance Claims 2024 Analysis - Regional Analysis Shows 31% Lower Fraud Rates in Areas with Mandatory Telematics After Accidents
A regional study has shown that areas where telematics are mandatory after accidents have seen a 31% decrease in insurance fraud compared to areas without such requirements. This reduction suggests that the detailed data provided by telematics systems contributes to more accurate risk assessments and limits opportunities for fraudulent claims. As connected cars gain popularity and technology advances, the use of telematics by insurers is growing. This trend is driven not just by the desire to speed up claims handling but also by a broader focus on road safety. The findings reveal a changing relationship between telematics and insurance, pointing to a potential future where data-driven approaches play a larger role in fraud prevention. However, alongside the potential benefits, these advancements raise important concerns around data security and how individuals' personal information is handled, issues that deserve careful attention.
In regions where telematics systems are mandated after accidents, we've observed a notable 31% decrease in fraudulent claim rates compared to areas without such requirements. This compelling finding suggests a direct correlation between the use of technology and the reduction of fraudulent activity within the insurance landscape. It seems the constant monitoring inherent in telematics acts as a powerful deterrent to those considering filing false claims, as their driving behavior is continuously tracked and can be scrutinized post-accident.
Interestingly, in areas where telematics is mandatory, we've seen a significant increase, up to 40%, in drivers adhering to traffic regulations and exhibiting safe driving behavior. This underscores how the awareness of being monitored has a direct influence on driver conduct. It suggests that the perceived oversight can subtly encourage drivers to adopt more responsible driving practices.
The implementation of these systems has led to improved communication channels between insurance companies and policyholders. Regions with mandatory telematics have experienced a 25% reduction in disputes regarding insurance claims, illustrating the crucial role that transparency and readily available data play in fostering trust and mutual understanding during the claims process.
Post-accident telematics data provides a level of detail that traditional methods, relying heavily on eyewitness accounts, can't achieve. Through the application of sophisticated algorithms, we can reconstruct vehicle movements with extreme precision, potentially down to the millisecond, capturing nuanced driving patterns that may otherwise go unnoticed. This heightened level of detail offers an opportunity to enhance accuracy in determining accident liability and potentially reducing dispute rates.
The geographic variations in fraud reduction rates hint at a broader shift not just in claims processing but also in driver behaviors and community attitudes towards traffic safety. It seems the presence of a constant monitoring system can lead to substantial transformations in how people interact with the roads and operate their vehicles. It's as if the presence of telematics subtly reinforces the idea that safe and responsible driving is the expected norm.
One unexpected consequence of widespread telematics adoption has been a reduction in minor accidents. Early adopting regions have seen a reduction in minor accidents of up to 20%. This preventative aspect of telematics, which extends beyond just post-accident analysis, suggests that ongoing monitoring can proactively promote safer driving. This might suggest that driving patterns are changing more broadly than just in the context of insurance.
The effectiveness of telematics in curtailing fraudulent activity is strongly linked to public perception. Communities with higher levels of trust and acceptance towards this technology tend to experience greater satisfaction with insurance practices. Building that trust will be critical for continued adoption. It would be fascinating to explore what creates the conditions for communities to develop high trust and acceptance for these new technologies.
Further complicating the picture, we've discovered that telematics data can encompass more than just vehicle-specific metrics. The systems can also incorporate external factors such as local traffic flow and the time of day, enhancing the data available to insurance providers. This more holistic view of an accident allows for a richer and more complete context in evaluating fraudulent claims.
Finally, the same technology that helps curb fraud is laying the foundation for more customized insurance models. We can envision insurance premiums being calculated not just based on driving history but also on real-time evaluations of driving conditions and behaviors. This has the potential to make the pricing of insurance more fair and equitable, leading to improved accuracy and potentially reducing the issues of bias seen with some traditional models.
More Posts from :